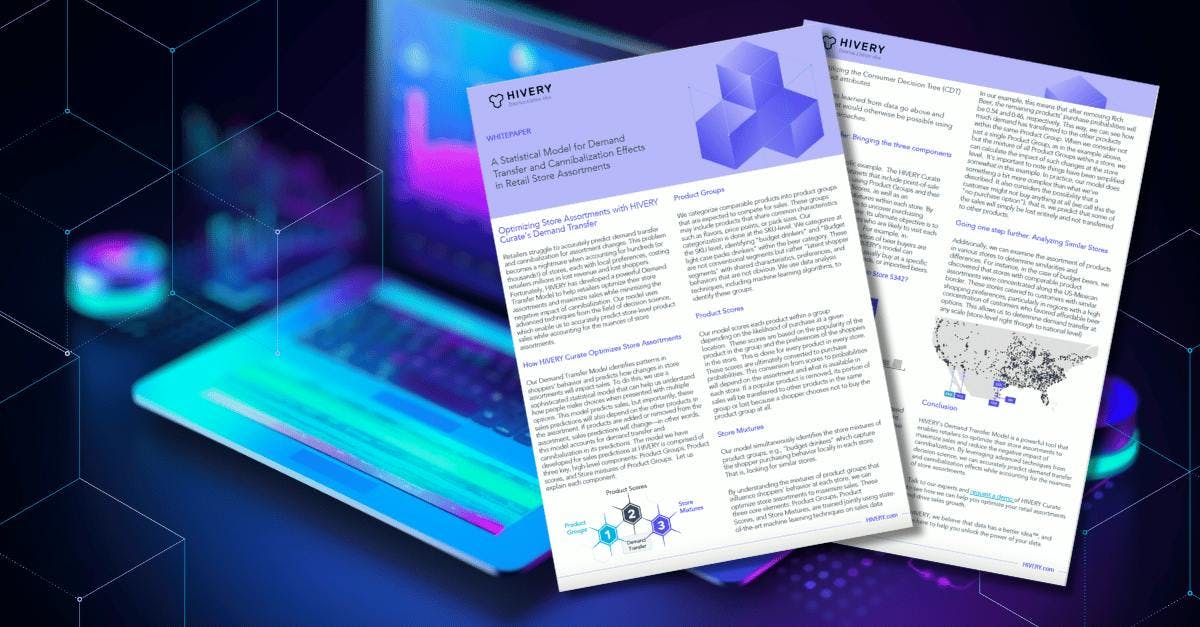
Unlocking the Secrets of Latent Shoppers: HIVERY's Demand Transfer Model Explained
The Demand Transfer Model is a tool that helps retailers understand how changes in their store's product assortment can impact sales. It uses data analysis techniques, including machine learning algorithms, to identify shopper behavior patterns and comparable group products into categories based on their competition for sales.
The model predicts the likelihood of a shopper buying a particular product in a store based on the popularity of that product in the category and the shoppers' preferences. It also considers the possibility that a shopper might not buy anything and predicts that some sales may be lost entirely and not transferred to other products.
By understanding the mix of product categories that influence shoppers' behavior at each store, the model helps retailers optimize their product assortments to maximize sales. For example, if a popular product is removed from a store, the model can predict how much demand will transfer to other products in the same category. This allows retailers to make informed decisions about their product assortment and ensure they meet their customers' needs and preferences.
One practical takeaway from this model is that retailers should regularly analyze their sales data and product assortment to identify patterns and trends in shopper behavior. This can help them decide what products to add or remove from their store's assortment to maximize sales.
Another practical takeaway is that retailers should consider the competition between products within categories when deciding their product assortment. By grouping comparable products into categories and analyzing the competition for sales within each category, retailers can ensure they offer a balanced assortment of products that meets the needs and preferences of their customers.
The critical point of difference with the HIVERY approach:
The key point of difference for the HIVERY model is that it is trained with store-level data, allowing for store-level data analysis and insights. This analysis is done for every SKU in every store, providing a comprehensive understanding of shopper behavior. Additionally, the model is dynamic and constantly learning as new data is used, generating new insights that can be applied to improve assortment strategy simulation. The model is also interactive, enabling users to change the assortment and see how demand is impacted in real time. This unique combination of store-level data analysis, dynamic learning, and interactivity makes HIVERY's Demand Transfer Model an essential tool for retailers looking to optimize their retail strategy and stay ahead of the competition.
Download Whitepaper
Related content you might be interested in:
- Maximizing Incrementality and Minimizing Cannibalization: How AI Builds Confidence and Trust in Category Strategies
- Progressive Grocer - Innovating Category Management: Assortment as a Service
- Do Current Assortment Optimization Methods Create Store Sacrifices?
- How To Get Assortment Space Contribution Index Optimized